LO³-ML
Low-Power Low-Memory Low-Cost ECG Signal Analysis using Machine Learning Algorithms
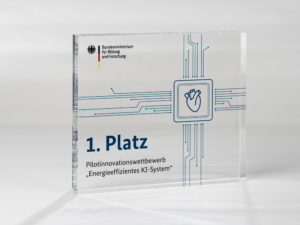
LO³-ML’s AI-chip earns a 1st Prize in a Germany-wide innovation contest for its high energy efficiency. https://www.iis.fraunhofer.de/en/pr/2021/20210319_ks_energy_saving_AI_chip.html
LO³-ML is a joint project between Fraunhofer IIS and Friedrich-Alexander-Universität Erlangen-Nürnberg. It is funded as part of the challenge for disruptive innovations in energy-efficient AI hardware initiated by the German Federal Ministry of Education and Research (BMBF). The goal of the project is to design a highly energy efficient ASIC (application-specific integrated circuit) for classification of atrial fibrillation in an ECG signal using neural networks by heavily optimizing neural network topologies and specifically adapting hardware architectures. To reduce the energy consumption to a minimum, Resistive Random Access Memory (RRAM) is applied as non-volatile, ternary parameter storage inside the chip.
More information about the innovation competition can be found here.
Project Partners
Friedrich-Alexander-Universität Erlangen-Nürnberg, Chair for Computer Architecture
Friedrich-Alexander-Universität Erlangen-Nürnberg, Institute for Electronics Engineering (LTE) https://www.lte.tf.fau.eu/
Fraunhofer Institute for Integrated Circuits IIS https://www.iis.fraunhofer.de/neuromorphic
Funding
German Federal Ministry of Education and Research (BMBF)
Project total
EUR 400 thousand
Project duration
October 1, 2019 – December 31, 2020
Project results
Completely designed mixed-signal digital/analog chip in 130 nm IHP process using RRAM cells. Post-layout simulation results in overall 270 nJ to classify any 2-minute-long ECG recording into “healthy” or “exhibits atrial fibrillation”. Designed chip is able to power down neural network accelerator during idle times and wake up quickly without significant energy consumption thanks to non-volatile RAMs. Our chip design can be applied to other time-series signals (voltages, audio, vibration etc.).
Publications
- M. Fritscher, J. Knödtel, M. Mallah, S. Pechmann, E. Perez-Bosch Quesada, T. Rizzi, C. Wenger, M. Reichenbach: „Mitigating the Effects of RRAM Process Variation on the Accuracy of Artificial Neural Networks“,SAMOS 21 Conference, Special Session on „Next Generation Computing“; https://samos-conference.com/wp/special-sessions/
- Reiser, Daniel; Reichel, Peter; Pechmann, Stefan; Mallah, Maen; Oppelt, Maximilian; Hagelauer, Amelie; Breiling, Marco; Fey, Dietmar; Reichenbach, Marc: „A Low Power Hardware Architecture for Embedded Classification Tasks using Neural Networks evaluated at the Example of an AFIB Detection ASIC“,IEEE Transactions on Computers (submitted)
- Markus Fritscher , Johannes Knödtel , Daniel Reiser, Maen Mallah, Stefan Pechmann , Dietmar Fey, Marc Reichenbach: „Simulating large neural networks embedding MLC RRAM as weight storage considering device variations“, 12th IEEE Latin America Symposium on Circuits and System, Arequipa, Perú, 21.-25. Februar 2021
- Knödtel, Johannes; Fritscher, Markus; Fey, Dietmar; Reichenbach, Marc; Reiser, Daniel; Breiling, Marco: „ A Model-to-Circuit Compiler for Evaluation of DNN Accelerators based on Systolic Arrays and Multibit Emerging Memories”, MOCAST 2020 – 9th International Conference on Modern Circuits and Systems Technologies (MOCAST) on Electronics and Communications
- Marco Breiling, Marc Reichenbach, and Peter Reichel: „AI Goes Ultra-Low-Power (Teil 1/2)“, Zeitschrift Elektronik xx/2021 (accepted)
- Breiling, Marco; Kundu, Bijoy; Reichenbach, Marc: „E=AI2“, ERCIM News 125, Special Issue on Brain-inspired Computing, April 2021
Funded by German Federal Ministry of Education and Research (BMBF) under grants 16ES1143 and 16ES1142K.